Ever wondered if AI has an ulterior motive with PPC campaigns, like it just wants to show off its algorithmic muscles? Spoiler alert: it does. But rather than plotting world domination, it’s more interested in optimising your ad spend and maximising those clicks, impressions, and conversions. Here's how machine learning (ML) is revolutionising pay-per-click (PPC) advertising, turning us all into digital marketing superheroes without the need for a cape.
The marvel of AI in PPC lies in its ability to process and analyse gargantuan datasets at lightning speed, a task that would leave the most seasoned data analyst weeping into their spreadsheets. ML algorithms can predict which keywords are likely to drive traffic and conversions by evaluating historical data, current trends, and user behaviour patterns. For instance, instead of you painstakingly bidding on hundreds of keywords, AI automates this process, dynamically adjusting bids in real-time based on performance indicators. This not only boosts efficiency but also ensures that your ads appear at the right time to the right audience. Talk about a time-saver.
Incorporating machine learning into PPC campaigns means leveraging predictive analytics and natural language processing (NLP) to refine ad copy and targeting. Predictive analytics forecasts future clicks and conversions by analysing past performance data, seasonal trends, and even external factors like economic indicators. NLP, on the other hand, helps in understanding the context and sentiment behind search queries, allowing for more precise keyword targeting and ad copy that resonates with the audience's intent. This sophisticated targeting means you're not just throwing darts in the dark but hitting bullseyes more often.
Moreover, AI’s prowess in A/B testing can’t be understated. Traditional A/B testing can be painfully slow, requiring substantial traffic and time to determine which ad variation performs best. ML algorithms accelerate this process by continuously testing multiple variables such as headlines, images, and calls-to-action simultaneously. The result? Optimised ad creatives that are constantly evolving based on real-time feedback. No more guesswork, just data-driven decisions that enhance ad performance.
Let’s not forget the role of AI in audience segmentation. Machine learning algorithms can sift through user data to identify patterns and segment audiences more precisely than ever before. These segments can be based on a multitude of factors, including demographics, online behaviour, purchasing history, and even psychographic data. Such granular segmentation allows for hyper-targeted ad campaigns, ensuring that your message reaches those most likely to convert. So, if you're promoting your eCommerce SEO services or small business SEO, AI ensures your ads are seen by the right people at the right time.
One of the crowning achievements of AI in PPC is the enhancement of Google Ads and Facebook Ads performance through automated bid strategies. AI-driven bidding strategies such as Target CPA (Cost Per Acquisition), Target ROAS (Return on Ad Spend), and Enhanced CPC (Cost Per Click) optimise bids to achieve the best possible results within your budget. These strategies take into account a plethora of factors, from user device and location to time of day and historical performance, ensuring your bids are not just competitive but also economically sound.
Then there's the aspect of dynamic search ads (DSAs), where AI takes the reins to create ad headlines and landing pages based on the content of your website. This is particularly useful for large eCommerce sites where manually creating ads for every product is impractical. AI ensures that your ads are always relevant to the search queries, increasing the likelihood of clicks and conversions. It's like having a digital butler that knows exactly what your audience is looking for and serves it up on a silver platter.
AI’s influence also extends to fraud detection in PPC campaigns. Click fraud, where competitors or bots repeatedly click on your ads to deplete your budget, is a significant concern. Machine learning algorithms can detect unusual patterns and behaviours indicative of click fraud, enabling swift action to mitigate its impact. This ensures that your ad spend is protected and utilised effectively, rather than being wasted on fraudulent clicks.
In conclusion, AI and machine learning have transformed PPC campaigns from a manual, labour-intensive endeavour into a sophisticated, data-driven strategy. By automating bid management, enhancing ad targeting, optimising ad creatives, segmenting audiences, and preventing click fraud, AI ensures that your PPC campaigns are not only effective but also efficient. As we continue to embrace these technologies, the future of digital advertising looks brighter—and a lot more automated.
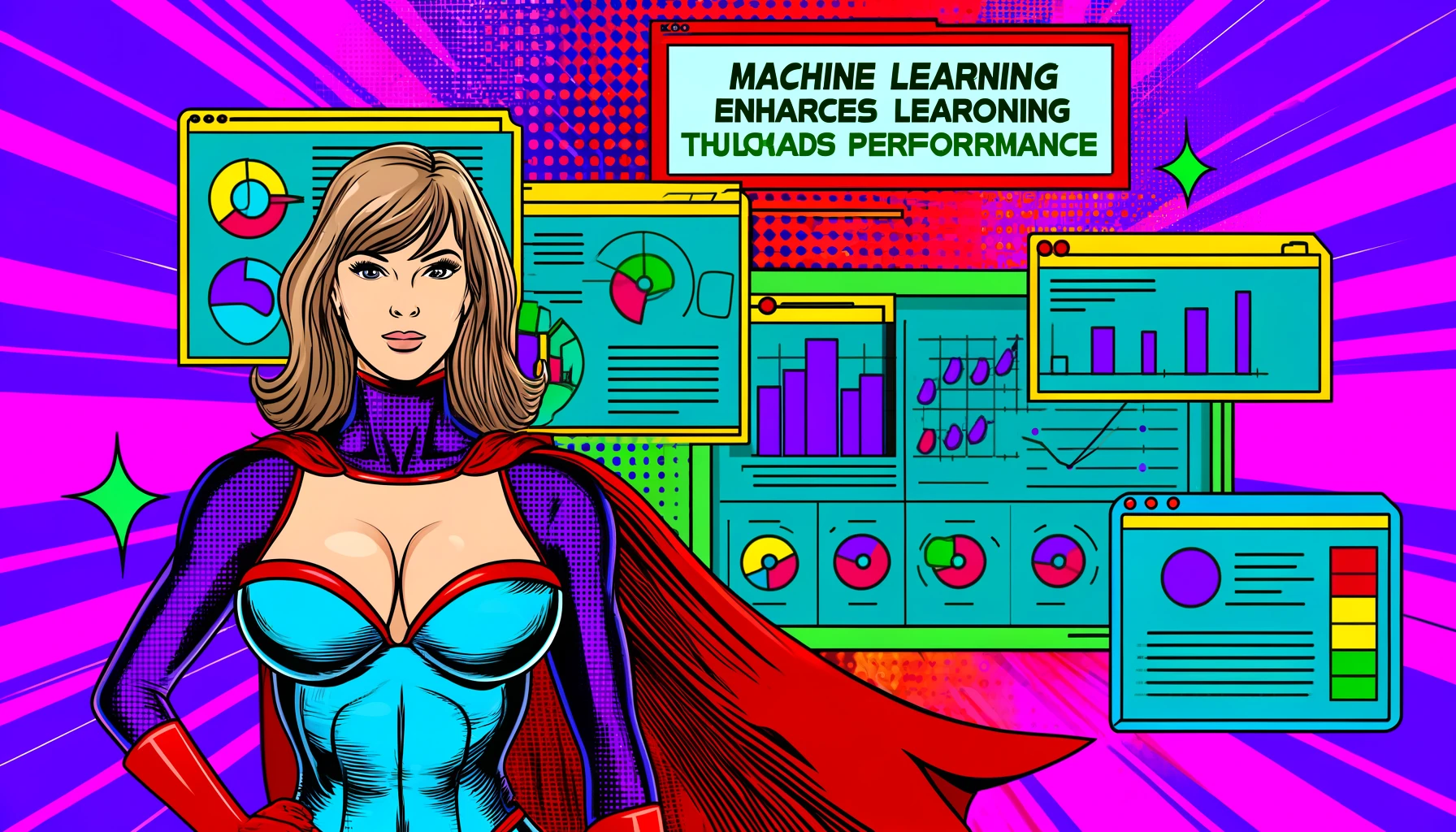
The Role of AI in Automating Ad Bidding Strategies
AI is revolutionising ad bidding strategies, turning what was once a manual, laborious task into a seamless, automated process. Imagine having an assistant who never sleeps, constantly tweaks your bids for optimal performance, and never complains about overtime. That's AI in ad bidding.
First off, let’s tackle how AI algorithms work their magic in bid automation. These algorithms analyse vast amounts of data, from user behaviour to market trends, to determine the optimal bid for each auction in real-time. Traditional bid strategies often relied on static rules and historical data, but AI changes the game by continuously learning and adapting to new information. For instance, it considers factors such as the time of day, user device, location, and even the weather to adjust bids dynamically. This ensures your ads are always competitively positioned without you having to lift a finger.
One of the standout features of AI-driven bidding is the use of predictive analytics. By examining past performance data and external factors, AI can forecast future outcomes and adjust bids accordingly. This isn’t just about staying ahead of the competition; it’s about anticipating user behaviour and maximising return on investment (ROI). Predictive analytics allows advertisers to allocate their budget more efficiently, ensuring higher conversion rates and lower cost per acquisition (CPA). For example, if historical data shows that conversion rates are higher on weekends for a particular product, the AI system will automatically increase bids during those times to capitalise on the trend.
AI also excels in identifying and targeting high-value audiences through advanced segmentation. Machine learning algorithms analyse user data to uncover patterns and segment audiences with surgical precision. This means your ads can be targeted to users most likely to convert, rather than casting a wide net and hoping for the best. If you're offering enterprise SEO services or technical SEO, AI ensures that your bids are directed towards decision-makers and businesses that are actively seeking these specialised services.
Moreover, AI-driven strategies like Target CPA, Target ROAS, and Enhanced CPC are designed to optimise bids in alignment with your specific goals. Target CPA focuses on acquiring conversions at a set cost per acquisition, adjusting bids to meet this target. Target ROAS aims to maximise the return on ad spend, dynamically bidding based on the predicted value of each click. Enhanced CPC, on the other hand, adjusts bids to increase the likelihood of conversions while staying within your budget. These strategies are continuously refined by AI, ensuring they remain effective even as market conditions change.
Dynamic bid adjustments based on real-time data are another feather in AI’s cap. Unlike static bidding, which requires constant manual intervention, AI-powered systems can instantly respond to changes in user behaviour, market trends, and competitive activity. This agility is crucial in maintaining ad performance and staying ahead of competitors. For instance, during a flash sale or a peak shopping season, AI can quickly ramp up bids to capture increased traffic and demand, ensuring your ads remain visible when it matters most.
AI’s role in preventing ad spend wastage through fraud detection is equally significant. Click fraud, where malicious actors artificially inflate clicks to drain your budget, has long plagued digital advertising. Machine learning algorithms can detect unusual patterns and behaviours that signify fraudulent activity, allowing for quick intervention and adjustment of bids to minimise financial loss. This ensures that your ad budget is spent on genuine clicks and conversions, not wasted on fraudulent activities.
Additionally, AI-powered tools like Google's Smart Bidding and Facebook’s Automated Rules have made sophisticated bid strategies accessible even to small businesses. These platforms leverage vast datasets and powerful algorithms to automate bid management, freeing advertisers to focus on strategy and creative development. Smart Bidding, for example, uses AI to optimise bids for conversions or conversion value across Google Ads campaigns, considering a wide range of contextual signals at auction time.
AI’s influence extends beyond just bid automation; it encompasses a holistic approach to campaign management. By integrating AI with other digital marketing tools, advertisers can create more cohesive and effective campaigns. For example, combining AI-driven bid strategies with AI-powered SEO content writing ensures that not only are your ads optimally positioned, but the content they lead to is also highly relevant and engaging.
In conclusion, the role of AI in automating ad bidding strategies is transformative. By leveraging real-time data analysis, predictive analytics, advanced segmentation, and fraud detection, AI ensures that ad bids are optimised for maximum performance and ROI. As AI technology continues to evolve, its capabilities in automating and enhancing ad bidding strategies will only grow, offering even greater efficiencies and effectiveness for digital advertisers.

Personalising Ad Content with Machine Learning Insights
Fancy a world where your ads speak directly to each potential customer like a digital mind-reader? Well, welcome to the machine learning (ML) era, where personalisation in ad content is taken to a whole new level. No more generic ads blasting into the void—ML ensures your ads are as relevant and engaging as a private conversation. Let’s explore how this tech wizardry works its magic.
First, let’s dissect how ML algorithms harness vast troves of data to personalise ad content. These algorithms analyse user behaviour, preferences, and interactions across various platforms to build detailed user profiles. It’s like having a digital Sherlock Holmes on your team, deducing interests and predicting future actions based on past behaviour. For instance, if a user frequently searches for SEO services and reads articles about digital marketing, ML can tailor ad content to showcase advanced SEO solutions and success stories. This hyper-targeted approach ensures that the ad resonates with the user's current needs and interests, leading to higher engagement and conversion rates.
Contextual understanding is another strength of ML. Natural Language Processing (NLP) enables algorithms to comprehend the context and sentiment behind user queries and interactions. This means your ad content isn’t just relevant; it’s also contextually appropriate. For instance, if a user’s search query indicates they are in the early stages of the purchasing journey, the ad might focus on educational content or introductory offers. Conversely, for users ready to buy, the ad could highlight competitive pricing and easy purchase options. This level of personalisation turns casual browsers into loyal customers by addressing their specific stage in the buying journey.
Dynamic content generation is where ML really shines. By utilising data-driven insights, ML can create personalised ad copy, images, and even videos on the fly. Imagine running an eCommerce campaign where the ad creative adapts based on the user’s browsing history. A user who recently viewed men’s shoes will see an ad featuring the latest styles in men’s footwear, while another user who looked at women’s accessories will see trending handbags and jewellery. This dynamic adaptation ensures that each user sees the most relevant products, boosting the likelihood of a click-through and eventual purchase.
Moreover, machine learning enhances retargeting efforts by understanding user behaviour patterns. Traditional retargeting can sometimes feel like digital stalking, showing the same ad repeatedly without considering the user’s evolving needs. ML refines this approach by analysing the frequency and recency of interactions, adjusting ad content to keep it fresh and engaging. For example, if a user has abandoned their shopping cart, an ML-driven retargeting ad might offer a discount or showcase related products to entice them back. This smart retargeting is more effective and less intrusive, increasing the chances of re-engagement.
Integrating ML with cross-channel marketing strategies is another game-changer. Users interact with brands across multiple touchpoints—social media, email, search engines, and websites. ML algorithms can track these interactions and deliver a consistent, personalised experience across all channels. For instance, a user who clicked on a Facebook Ad for your eCommerce SEO services might later receive a follow-up email with case studies and testimonials, reinforcing the ad’s message. This cohesive experience enhances brand recall and fosters a deeper connection with the audience.
Predictive analytics plays a crucial role in anticipating user needs and tailoring ad content accordingly. By analysing historical data and current trends, ML algorithms can predict what users are likely to want or need next. This foresight allows advertisers to create proactive ad campaigns that cater to these anticipated needs. For instance, if data shows a surge in interest for summer travel during early spring, ML can suggest creating ads for travel packages, sunscreen, and summer apparel, ensuring that your content is timely and relevant.
The personalisation prowess of ML extends to audience segmentation as well. By diving deep into user data, ML can identify micro-segments within your audience that share similar characteristics and behaviours. This granular segmentation allows for ultra-targeted ad campaigns. For example, a small business SEO provider can create tailored ads for different industries such as healthcare, retail, or finance, each highlighting specific benefits and success stories relevant to that sector. This level of detail ensures that your ad speaks directly to the unique pain points and aspirations of each segment.
Lastly, ML’s real-time optimisation capabilities mean that your ad content is continually refined based on performance data. This dynamic adjustment ensures that your ads remain effective in the face of changing user behaviour and market conditions. For instance, if an ad variant shows higher engagement among a particular demographic, ML can allocate more budget to that variant and tweak the content for even better results. This ongoing optimisation maximises ROI and ensures that your ad campaigns are always performing at their peak.
In conclusion, personalising ad content with machine learning insights is not just a technological advancement; it’s a paradigm shift in digital marketing. By leveraging data-driven insights, contextual understanding, dynamic content generation, smart retargeting, cross-channel integration, predictive analytics, advanced segmentation, and real-time optimisation, ML transforms generic ads into highly personalised, engaging experiences. This leads to higher engagement, better conversion rates, and ultimately, a more loyal customer base.
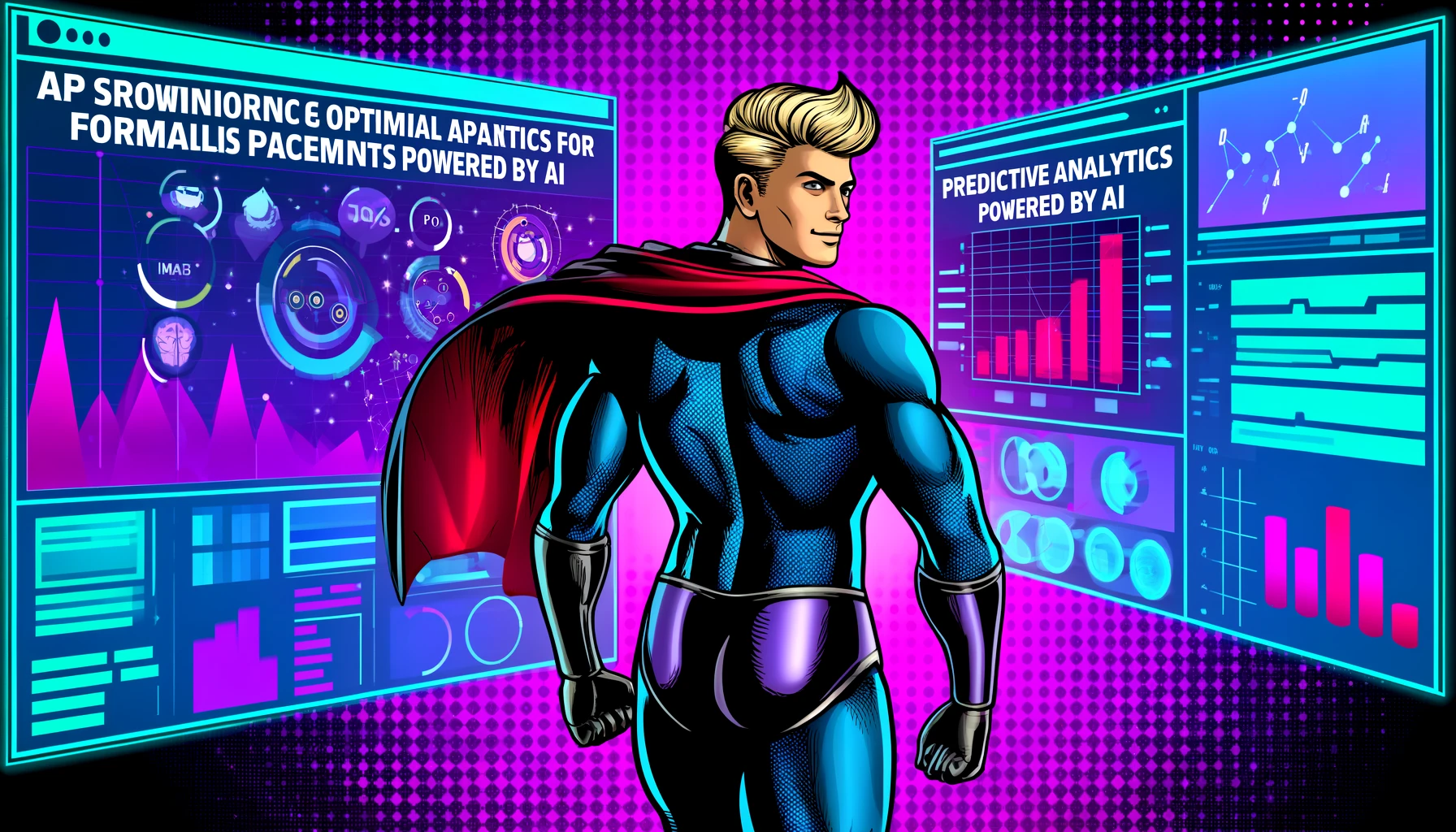
Leveraging Predictive Analytics for Optimal Ad Placement
Imagine your ads playing the perfect game of darts, hitting the bullseye every time. That’s the power of predictive analytics in ad placement, a game-changer that makes traditional methods look like a shot in the dark. With predictive analytics, you're not just reacting to past data; you’re anticipating future actions. Let's delve into how this technology works and why it's crucial for achieving optimal ad placement.
Predictive analytics uses machine learning (ML) algorithms and statistical models to analyse historical data and predict future trends. This technology can forecast which ad placements will yield the highest engagement and conversions by examining patterns and behaviours from past campaigns. For instance, an algorithm might discover that certain times of day or specific types of websites consistently generate higher click-through rates (CTR). Armed with this insight, advertisers can strategically place ads to capitalise on these peak periods and locations, ensuring maximum impact.
The power of predictive analytics lies in its ability to process and interpret vast amounts of data quickly and accurately. It evaluates numerous variables, such as user demographics, browsing behaviour, device type, and even external factors like seasonal trends and economic indicators. This comprehensive analysis enables the creation of highly targeted ad campaigns that resonate with specific audience segments. For example, if data shows that users interested in enterprise SEO services are more active on LinkedIn during weekday mornings, predictive analytics can allocate a larger portion of the ad budget to these times and platforms.
One of the most significant advantages of predictive analytics is its capacity to enhance real-time bidding (RTB) strategies. In RTB, advertisers bid on ad impressions in real-time, with the highest bidder getting their ad displayed. Predictive models can estimate the value of each impression based on historical performance and current context, allowing advertisers to make more informed bidding decisions. This ensures that your ads are placed in front of the right audience at the right time, maximising the return on ad spend (ROAS).
Moreover, predictive analytics excels in refining audience segmentation. By analysing user data, ML algorithms can identify micro-segments within your target audience that share similar characteristics and behaviours. This granular segmentation allows for more precise ad placements. For example, a campaign for technical SEO services might target IT professionals who have recently engaged with content on website optimisation. These highly specific segments ensure that your ads are shown to users who are most likely to be interested in your services, increasing the likelihood of conversions.
Cross-channel marketing also benefits immensely from predictive analytics. Users interact with brands across multiple platforms—social media, search engines, email, and websites. Predictive models can track these interactions and determine the most effective channels and times for ad placements. For instance, if data indicates that users who visit your site via Google Ads also respond well to follow-up emails, you can integrate these channels into a cohesive strategy. This cross-channel synergy ensures that your ads are seen by users at multiple touchpoints, reinforcing your message and driving higher engagement.
The dynamic nature of predictive analytics means that it continuously learns and adapts from new data. This real-time learning capability ensures that your ad placements remain optimal even as user behaviour and market conditions evolve. For instance, if an ML model detects a sudden increase in engagement from a particular demographic, it can adjust ad placements to target this group more aggressively. This agility is crucial in maintaining the effectiveness of ad campaigns over time.
Furthermore, predictive analytics plays a pivotal role in preventing ad fraud. Click fraud, where bots or malicious actors generate fake clicks, can significantly drain your ad budget. Predictive models can identify unusual patterns and behaviours indicative of fraud, allowing for quick intervention and adjustment of ad placements. This ensures that your ad spend is protected and used efficiently, targeting genuine users rather than fraudulent clicks.
Incorporating predictive analytics into your ad strategy also enhances A/B testing. Traditional A/B testing can be time-consuming and limited in scope, but predictive models can run multiple tests simultaneously, evaluating different ad creatives, placements, and audience segments. This rapid testing and optimisation process means that the most effective ad variations are quickly identified and scaled, improving overall campaign performance.
To summarise, leveraging predictive analytics for ad placement transforms how advertisers approach digital marketing. By analysing historical data, predicting future trends, and continuously learning and adapting, predictive models ensure that ads are placed optimally to achieve the highest engagement and conversions. Whether it's refining audience segmentation, enhancing real-time bidding, or preventing ad fraud, predictive analytics offers a powerful tool for maximising the effectiveness of ad campaigns.

Real-Time Ad Performance Tracking with AI
Forget about waiting for weekly reports or sifting through endless data manually—AI-powered real-time ad performance tracking is here to make your life easier and your campaigns more effective. Let’s delve into how AI revolutionises ad performance tracking, ensuring your campaigns are always on the right track.
Real-time ad performance tracking is essentially having a hyper-attentive digital assistant monitoring every click, impression, and conversion as they happen. AI algorithms process vast amounts of data instantaneously, offering insights that allow marketers to tweak and optimise campaigns on the fly. No more waiting for end-of-month reports to see how your ads are doing; with AI, you get immediate feedback.
One of the standout features of AI in ad performance tracking is its ability to provide detailed, actionable insights. Traditional analytics tools might show you the basics—click-through rates (CTR), conversion rates, and cost per click (CPC). AI, however, goes several steps further by analysing patterns and predicting trends. For example, if your Google Ads campaign sees a sudden spike in CTR, AI can identify the cause, whether it's a change in keyword relevance, an uptick in user interest, or a competitor's activity. This level of detail allows you to make informed decisions quickly, adjusting bids, ad copy, or targeting parameters to capitalise on emerging opportunities.
AI-driven tools excel in multi-channel performance tracking. Users interact with ads across various platforms—search engines, social media, email, and websites. AI aggregates data from these diverse sources into a cohesive, real-time dashboard. This unified view is crucial for understanding how different channels contribute to overall campaign success and how they interact with each other. For instance, if users frequently engage with your Facebook Ads but convert more often after visiting your website, AI can highlight this journey, allowing you to adjust your strategy to better nurture these leads through the sales funnel.
Another advantage of real-time tracking with AI is its predictive analytics capability. By analysing current and historical data, AI can forecast future performance trends, enabling proactive adjustments. Suppose your eCommerce SEO services campaign is trending positively on weekends. In that case, AI can suggest increasing your ad spend during these peak periods while pulling back during slower times. This predictive foresight ensures that your budget is always optimally allocated, maximising return on investment (ROI).
AI's ability to personalise insights is another game-changer. Every campaign is unique, with its own set of goals, target audience, and performance metrics. AI customises tracking and reporting to focus on the KPIs that matter most to your specific campaign. Whether it's tracking the performance of a new product launch or measuring the impact of a seasonal promotion, AI tailors its analysis to provide the most relevant insights. This personalised approach ensures that you're always focusing on the data that will drive the most significant improvements.
Real-time performance tracking also enhances A/B testing efficiency. Traditional A/B testing can be a slow, cumbersome process, often requiring significant traffic and time to determine which ad variation performs best. AI accelerates this process by continuously monitoring and comparing multiple ad versions, providing instant feedback on which elements resonate most with your audience. This rapid iteration allows for swift optimisation, ensuring that your ads are always as effective as possible.
One often overlooked aspect of AI in real-time tracking is its role in anomaly detection. Campaigns don't always go as planned; sudden drops in performance or unexpected spikes can indicate issues such as ad fatigue, technical glitches, or even click fraud. AI algorithms excel at detecting these anomalies, alerting you immediately so you can investigate and rectify the problem before it escalates. This proactive monitoring safeguards your ad spend and maintains campaign efficiency.
Moreover, AI-powered real-time tracking enhances collaboration among marketing teams. By providing a centralised, real-time dashboard accessible to all stakeholders, AI fosters a more collaborative and informed decision-making process. Team members can share insights, discuss strategies, and implement changes without waiting for periodic reports, ensuring that everyone is on the same page and working towards the same goals.
The integration of AI with other marketing tools further amplifies its impact. For instance, combining AI-driven performance tracking with AI-powered SEO content writing ensures that your ad campaigns are not only monitored and optimised but also supported by high-quality, relevant content. This holistic approach maximises the effectiveness of your entire digital marketing strategy, creating a seamless and compelling user experience from ad click to conversion.
In conclusion, real-time ad performance tracking with AI transforms how advertisers monitor and optimise their campaigns. By providing immediate, detailed insights, predictive analytics, personalised tracking, and anomaly detection, AI ensures that your ad campaigns are always performing at their best. This dynamic, data-driven approach not only enhances efficiency but also drives higher engagement and conversions, making AI an indispensable tool for modern digital marketers.

Enhancing Targeting Precision with Machine Learning Algorithms
Let's talk precision—sharpening your ad targeting to a laser focus that makes traditional methods look like aiming with a blindfold. Machine learning (ML) algorithms are at the heart of this transformation, turning vast amounts of data into actionable insights. This isn't just about hitting your audience; it's about hitting the right part of your audience at the right time with the right message. So, let's unpack how ML enhances targeting precision in digital advertising.
At its core, machine learning analyses vast datasets to uncover patterns and insights that are imperceptible to the human eye. By leveraging these insights, ML can predict which segments of your audience are most likely to engage with your ads. This predictive capability allows advertisers to go beyond basic demographic targeting, diving deep into behavioural and psychographic data. For instance, an ML model can identify that users who engage with enterprise SEO services content are not just interested in SEO, but specifically in scalable, high-impact solutions. This level of granularity ensures your ad messaging resonates with their specific needs and pain points.
Dynamic audience segmentation is another area where ML excels. Traditional segmentation might categorise users by age, gender, or location, but ML algorithms can create micro-segments based on a multitude of variables such as browsing behaviour, purchase history, and social media interactions. For example, a campaign for technical SEO services can target not just IT professionals, but those who have recently engaged with content about website speed optimisation and mobile-first indexing. These nuanced segments allow for hyper-targeted campaigns that significantly increase engagement and conversion rates.
One of the most powerful applications of ML in targeting precision is in lookalike modelling. This involves identifying new potential customers who share similar characteristics and behaviours with your existing high-value customers. ML algorithms analyse your current customer data to build a profile of what makes them valuable—such as purchase frequency, average order value, and engagement metrics. They then scour broader datasets to find new users who match this profile, effectively expanding your reach to likely converters without the guesswork. If your high-value customers are avid consumers of Google Ads strategies, ML will find more users with similar online behaviours and interests.
Real-time data processing and adaptation is another crucial advantage of ML. As user behaviour changes, so too do the algorithms' predictions and segmentations. This real-time adaptability ensures that your targeting remains relevant and effective. For example, if there's a sudden trend in users seeking SEO content writing due to a new algorithm update from Google, ML algorithms will detect this shift and adjust your targeting strategy accordingly, ensuring your ads stay ahead of the curve.
Personalisation at scale is made possible through ML, allowing for tailored ad experiences that were previously unimaginable. By analysing individual user data, ML can personalise ad content in real-time, ensuring that each user sees the most relevant message. For instance, a user who frequently searches for tips on optimising eCommerce websites might see ads highlighting eCommerce SEO services with specific case studies and success stories. This level of personalisation significantly boosts engagement and conversion rates by addressing the user's immediate needs and interests.
AI-powered predictive analytics enhance targeting precision by forecasting future user behaviours based on historical data. This foresight allows advertisers to anticipate user needs and tailor their campaigns accordingly. For example, if data indicates that interest in holiday travel spikes in early November, an AI system can start promoting travel deals and related products in late October, capitalising on this trend before competitors catch on. This proactive approach ensures that your ads are always timely and relevant, increasing their effectiveness.
Moreover, integrating ML with programmatic advertising platforms enhances targeting precision by automating the ad buying process based on real-time data. Programmatic advertising uses ML algorithms to decide which ads to buy and how much to pay for them, based on the predicted performance of each ad impression. This automated bidding process ensures that your ads are placed in front of the most relevant audience segments, at the best possible times, maximising the efficiency of your ad spend.
Cross-channel targeting precision is another area where ML shines. Users interact with brands across multiple touchpoints—social media, email, search engines, and websites. ML algorithms track these interactions and create a unified user profile, enabling consistent and precise targeting across all channels. For instance, a user who interacts with your brand on Instagram might see a retargeted ad on Facebook, followed by a personalised email offer. This seamless, cross-channel experience enhances user engagement and drives higher conversion rates.
ML also plays a vital role in mitigating ad fatigue by rotating ad creatives and optimising delivery times. Ad fatigue occurs when users see the same ad too often, leading to decreased engagement. ML algorithms can detect when a particular ad is losing effectiveness and adjust the ad mix to keep content fresh and engaging. For example, if data shows that a certain audience segment has seen an ad multiple times without converting, the algorithm might introduce a new ad variation or change the delivery time to recapture their interest.
In conclusion, machine learning significantly enhances targeting precision in digital advertising. By leveraging advanced data analysis, dynamic audience segmentation, real-time adaptability, and personalised ad experiences, ML ensures that your ads are always relevant, timely, and effective. This sophisticated approach not only maximises engagement and conversion rates but also optimises ad spend, making ML an indispensable tool for modern digital marketers.
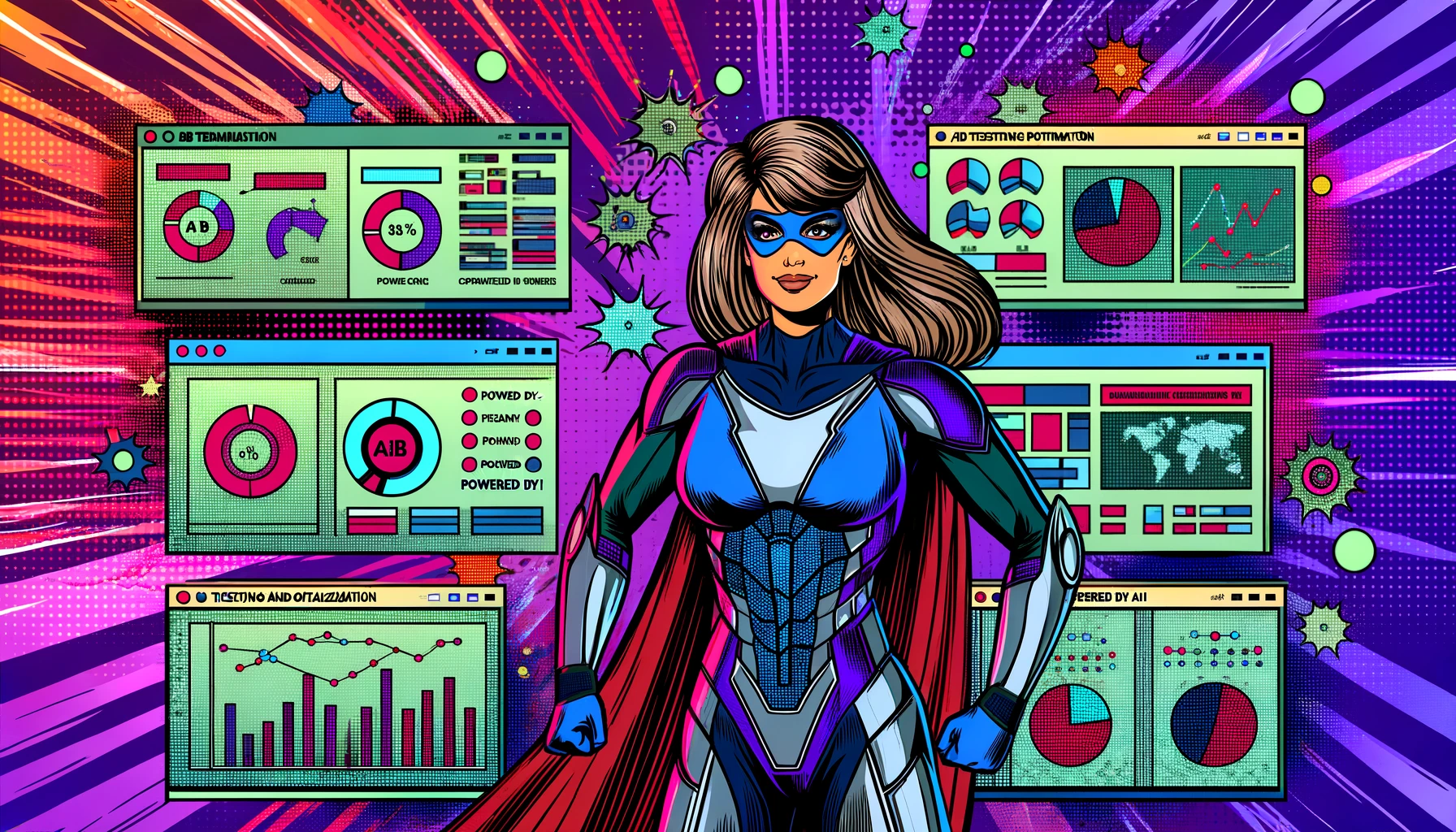
A/B Testing and Optimisation: The AI Advantage
Ever feel like A/B testing is akin to rolling the dice, hoping your latest ad tweak hits the jackpot? With AI, we can swap that dice roll for a precision-guided missile. AI-driven A/B testing isn't just about making educated guesses; it's about leveraging vast amounts of data to continually refine and optimise in real-time. Let’s explore how AI turbocharges A/B testing and optimisation, turning it from a time-consuming task into an efficient, dynamic process.
Traditional A/B testing involves running two variations of an ad to see which performs better, based on metrics like click-through rates (CTR) or conversions. While effective, this method can be slow and limited in scope. Enter AI, which enhances A/B testing through continuous, real-time analysis and adaptation. AI algorithms can run multiple test variations simultaneously, analysing performance data on the fly and adjusting variables to maximise results. For example, instead of testing two headlines, AI can simultaneously test dozens, evaluating which combinations of headlines, images, and calls-to-action drive the highest engagement.
One of the significant advantages of AI in A/B testing is its ability to handle multivariate testing effortlessly. Traditional A/B testing might struggle with more than two or three variations due to the exponential increase in complexity. AI, however, thrives on complexity. It can test numerous combinations of different elements—headlines, images, ad copy, and more—identifying the best-performing combinations quickly. This means you can optimise your Facebook Ads or Google Ads campaigns at a granular level, ensuring every element contributes to the ad’s success.
AI’s predictive analytics capabilities also play a crucial role in A/B testing. By analysing historical data and current trends, AI can predict which ad variations are likely to perform best even before the testing phase is complete. This predictive insight allows for preemptive optimisation, where less promising variations are phased out early, and more resources are allocated to high-performing ones. For instance, if an algorithm predicts that a particular ad variant will achieve a higher conversion rate based on user engagement patterns, you can quickly pivot and focus on that variant, saving time and budget.
Moreover, AI excels in segmenting audiences for more precise testing. Traditional A/B testing often treats the audience as a monolithic group, but AI can identify micro-segments based on various factors such as demographics, behaviour, and past interactions. For example, an eCommerce SEO campaign might reveal that different headlines work better for different audience segments—technical buyers might respond more to data-driven claims, while creative professionals might prefer emotional appeals. AI-driven segmentation ensures that each audience segment sees the most effective ad variant, maximising overall campaign performance.
Real-time feedback and adaptation are where AI truly shines. Traditional A/B tests run for a set period, with analysis and adjustments made afterward. AI, however, enables continuous testing and optimisation. As soon as data comes in, AI algorithms analyse it and adjust the campaign parameters in real-time. This agility ensures that your ads are always optimised for current conditions, significantly boosting efficiency and effectiveness. For instance, if a particular ad copy starts underperforming, AI can tweak or replace it immediately, maintaining high engagement levels throughout the campaign.
AI-driven A/B testing also enhances personalisation. By continuously learning from user interactions, AI can personalise ad variations to individual users. For example, if a user has shown a preference for certain product features in the past, AI can tailor the ad content to highlight those features. This level of personalisation not only improves user experience but also drives higher conversion rates. Imagine promoting your SEO content writing services with different messages—one focusing on technical expertise for a tech-savvy audience and another on creativity for a marketing-focused audience. AI ensures each user sees the most relevant ad, maximising engagement.
Another critical benefit of AI in A/B testing is its ability to minimise ad fatigue. Ad fatigue occurs when users see the same ad too often, leading to decreased engagement. AI algorithms can detect signs of ad fatigue early and rotate ad variations to keep the content fresh and engaging. For instance, if an ad for technical SEO starts to show diminishing returns, AI can introduce new creative elements or switch to a different ad variant to maintain user interest.
The integration of AI with cross-channel marketing strategies further amplifies its impact. Users interact with ads across multiple platforms—social media, search engines, email, and websites. AI can track these interactions and provide insights on how different ad variations perform across channels. This holistic view ensures that your A/B testing efforts are not siloed but contribute to a cohesive and effective overall strategy. For example, an ad that performs well on Instagram might inform adjustments to similar ads on Facebook or your website, ensuring consistency and optimisation across all touchpoints.
In summary, AI transforms A/B testing and optimisation from a static, laborious process into a dynamic, continuous cycle of improvement. By leveraging real-time data analysis, predictive analytics, advanced segmentation, and personalisation, AI ensures that your ad campaigns are always performing at their peak. This sophisticated approach not only enhances engagement and conversion rates but also optimises ad spend, making AI an invaluable tool for modern digital marketers.

Future Trends: The Evolution of AI in PPC Campaigns
Imagine AI in PPC campaigns not just as a tool, but as a strategic partner that evolves continuously, transforming the landscape with each passing year. The future of AI in pay-per-click (PPC) advertising is set to be marked by unprecedented advancements, making current strategies look quaint by comparison. Let’s explore the emerging trends and innovations poised to redefine PPC campaigns.
First, let’s discuss hyper-personalisation. As AI technologies become more sophisticated, the ability to create highly personalised ad experiences will reach new heights. Future AI systems will analyse vast arrays of data points, from purchase history and browsing behaviour to social media interactions and even real-time emotional states. This will enable the creation of ad content tailored to the unique preferences and circumstances of individual users. For instance, if a user shows a preference for eco-friendly products, AI can dynamically generate ads highlighting the sustainability features of your offerings. This level of personalisation will significantly enhance user engagement and conversion rates.
Voice search optimisation is another critical area where AI will make significant strides. With the growing prevalence of voice-activated devices like Amazon’s Alexa and Google Assistant, voice search is becoming a dominant mode of interaction. Future PPC campaigns will need to adapt by optimising for voice search queries, which are typically longer and more conversational than text searches. AI will play a crucial role in understanding and predicting these queries, ensuring that ads are effectively targeted and relevant. Imagine your ad for local SEO services appearing precisely when someone asks their device, “Where can I find the best SEO services near me?”
Predictive audience targeting will also see substantial advancements. Current AI systems can already predict user behaviour to some extent, but future developments will take this capability to a whole new level. Advanced predictive analytics will allow AI to anticipate user needs and preferences with remarkable accuracy, even before users themselves are aware of them. This foresight will enable advertisers to create proactive campaigns that meet users at the perfect moment in their decision-making journey. For example, AI might detect a user’s growing interest in digital marketing strategies and preemptively serve ads for SEO content writing just as they start exploring related topics.
The integration of AI with augmented reality (AR) and virtual reality (VR) technologies will revolutionise ad engagement. As AR and VR become more mainstream, AI will help create immersive, interactive ad experiences that engage users in entirely new ways. For instance, an AI-driven AR ad could allow users to visualise how a piece of furniture would look in their home or how a new pair of glasses would fit on their face. This interactive element not only enhances user experience but also drives higher engagement and conversion rates.
AI’s role in fraud detection and prevention will continue to evolve, becoming even more sophisticated. Click fraud and ad fraud remain significant challenges in digital advertising, costing advertisers billions each year. Future AI systems will employ advanced machine learning algorithms to detect and mitigate fraud in real-time, ensuring that ad spend is used efficiently and effectively. These systems will be capable of identifying complex fraud patterns that elude current detection methods, providing a robust defence against increasingly sophisticated fraudulent activities.
Dynamic creative optimisation (DCO) will become a standard feature in AI-driven PPC campaigns. DCO allows for the automatic generation and testing of ad creatives based on real-time data. Future AI systems will enhance this capability, enabling the creation of highly customised ads that adapt to user behaviour and preferences in real-time. For example, an AI system might generate different ad versions for a user interested in Google Ads based on their past interactions, presenting one version focusing on advanced analytics and another on cost-efficiency, depending on their previous engagement.
The convergence of AI with other emerging technologies, such as blockchain, will also shape the future of PPC campaigns. Blockchain’s transparency and security features can complement AI’s analytical prowess, creating a more trustworthy and efficient ad ecosystem. For instance, blockchain can verify the authenticity of ad impressions and clicks, while AI analyses the performance data to optimise ad placements and targeting strategies. This synergy will enhance the overall effectiveness and integrity of PPC campaigns.
Enhanced sentiment analysis will allow AI to gauge user emotions more accurately, tailoring ad content to resonate on a deeper emotional level. Future AI systems will not only analyse text but also voice intonations and facial expressions in video interactions to understand user sentiment. This will enable advertisers to create emotionally intelligent ads that connect with users on a more personal level, driving higher engagement and loyalty.
Finally, the evolution of AI will bring about more sophisticated cross-channel marketing strategies. Future AI systems will seamlessly integrate data from various channels—social media, email, search engines, and websites—to create a unified view of user behaviour. This holistic approach will enable more coherent and effective ad campaigns, ensuring that users receive consistent and relevant messages across all touchpoints. For example, an AI-driven campaign might coordinate a Facebook Ad with follow-up emails and targeted search ads, creating a seamless user journey that maximises conversion opportunities.
In conclusion, the future of AI in PPC campaigns is incredibly promising, with advancements set to enhance personalisation, voice search optimisation, predictive targeting, AR/VR integration, fraud prevention, dynamic creative optimisation, blockchain synergy, sentiment analysis, and cross-channel marketing. These innovations will not only improve the effectiveness and efficiency of PPC campaigns but also create more engaging and meaningful user experiences. As AI continues to evolve, it will undoubtedly play a pivotal role in shaping the future of digital advertising.
For further reading and expert insights on the future trends of AI in PPC campaigns, consider these sources: